Sticky Postings
By fabric | ch
-----
As we continue to lack a decent search engine on this blog and as we don't use a "tag cloud" ... This post could help navigate through the updated content on | rblg (as of 09.2023), via all its tags!
FIND BELOW ALL THE TAGS THAT CAN BE USED TO NAVIGATE IN THE CONTENTS OF | RBLG BLOG:
(to be seen just below if you're navigating on the blog's html pages or here for rss readers)
--
Note that we had to hit the "pause" button on our reblogging activities a while ago (mainly because we ran out of time, but also because we received complaints from a major image stock company about some images that were displayed on | rblg, an activity that we felt was still "fair use" - we've never made any money or advertised on this site).
Nevertheless, we continue to publish from time to time information on the activities of fabric | ch, or content directly related to its work (documentation).
Monday, February 06. 2017
Note: following the two previous posts about algorythms and bots ("how do they ... ?), here comes a third one.
Slighty different and not really dedicated to bots per se, but which could be considered as related to "machinic intelligence" nonetheless. This time it concerns techniques and algoritms developed to understand the brain (BRAIN initiative, or in Europe the competing Blue Brain Project).
In a funny reversal, scientists applied techniques and algorythms developed to track human intelligence patterns based on data sets to the computer itself. How do a simple chip "compute information"? And the results are surprising: the computer doesn't understand how the computer "thinks" (or rather works in this case)!
This to confirm that the brain is certainly not a computer (made out of flesh)...
Via MIT Technology Review
-----
Neuroscience Can’t Explain How an Atari Works
By Jamie Condlife
When you apply tools used to analyze the human brain to a computer chip that plays Donkey Kong, can they reveal how the hardware works?
Many research schemes, such as the U.S. government’s BRAIN initiative, are seeking to build huge and detailed data sets that describe how cells and neural circuits are assembled. The hope is that using algorithms to analyze the data will help scientists understand how the brain works.
But those kind of data sets don’t yet exist. So Eric Jonas of the University of California, Berkeley, and Konrad Kording from the Rehabilitation Institute of Chicago and Northwestern University wondered if they could use their analytical software to work out how a simpler system worked.
They settled on the iconic MOS 6502 microchip, which was found inside the Apple I, the Commodore 64, and the Atari Video Game System. Unlike the brain, this slab of silicon is built by humans and fully understood, down to the last transistor.
The researchers wanted to see how accurately their software could describe its activity. Their idea: have the chip run different games—including Donkey Kong, Space Invaders, and Pitfall, which have already been mastered by some AIs—and capture the behavior of every single transistor as it did so (creating about 1.5 GB per second of data in the process). Then they would turn their analytical tools loose on the data to see if they could explain how the microchip actually works.
For instance, they used algorithms that could probe the structure of the chip—essentially the electronic equivalent of a connectome of the brain—to establish the function of each area. While the analysis could determine that different transistors played different roles, the researchers write in PLOS Computational Biology, the results “still cannot get anywhere near an understanding of the way the processor really works.”
Elsewhere, Jonas and Kording removed a transistor from the microchip to find out what happened to the game it was running—analogous to so-called lesion studies where behavior is compared before and after the removal of part of the brain. While the removal of some transistors stopped the game from running, the analysis was unable to explain why that was the case.
In these and other analyses, the approaches provided interesting results—but not enough detail to confidently describe how the microchip worked. “While some of the results give interesting hints as to what might be going on,” explains Jonas, “the gulf between what constitutes ‘real understanding’ of the processor and what we can discover with these techniques was surprising.”
It’s worth noting that chips and brains are rather different: synapses work differently from logic gates, for instance, and the brain doesn’t distinguish between software and hardware like a computer. Still, the results do, according to the researchers, highlight some considerations for establishing brain understanding from huge, detailed data sets.
First, simply amassing a handful of high-quality data sets of the brains may not be enough for us to make sense of neural processes. Second, without many detailed data sets to analyze just yet, neuroscientists ought to remain aware that their tools may provide results that don’t fully describe the brain’s function.
As for the question of whether neuroscience can explain how an Atari works? At the moment, not really.
(Read more: “Google's AI Masters Space Invaders (But It Still Stinks at Pac-Man),” “Government Seeks High-Fidelity ‘Brain-Computer’ Interface”)
Saturday, July 06. 2013
Via MIT Technology Review
-----
By
Loren M. Frank
Enhancing the flow of information through the brain could be crucial to making neuroprosthetics practical.
The abilities to learn, remember, evaluate, and decide are central to who we are and how we live. Damage to or dysfunction of the brain circuitry that supports these functions can be devastating, leading to Alzheimer’s, schizophrenia, PTSD, or many other disorders. Current treatments, which are drug-based or behavioral, have limited efficacy in treating these problems. There is a pressing need for something more effective.
One promising approach is to build an interactive device to help the brain learn, remember, evaluate, and decide. One might, for example, construct a system that would identify patterns of brain activity tied to particular experiences and then, when called upon, impose those patterns on the brain. Ted Berger, Sam Deadwyler, Robert Hampsom, and colleagues have used this approach (see “Memory Implants”). They are able to identify and then impose, via electrical stimulation, specific patterns of brain activity that improve a rat’s performance in a memory task. They have also shown that in monkeys stimulation can help the animal perform a task where it must remember a particular item.
Their ability to improve performance is impressive. However, there are fundamental limitations to an approach where the desired neural pattern must be known and then imposed. The animals used in their studies were trained to do a single task for weeks or months and the stimulation was customized to produce the right outcome for that task. This is only feasible for a few well-learned experiences in a predictable and constrained environment.
New and complex experiences engage large numbers of neurons scattered across multiple brain regions. These individual neurons are physically adjacent to other neurons that contribute to other memories, so selectively stimulating the right neurons is difficult if not impossible. And to make matters even more challenging, the set of neurons involved in storing a particular memory can evolve as that memory is processed in the brain. As a result, imposing the right patterns for all desired experiences, both past and future, requires technology far beyond what is possible today.
I believe the answer to be an alternative approach based on enhancing flows of information through the brain. The importance of information flow can be appreciated when we consider how the brain makes and uses memories. During learning, information from the outside world drives brain activity and changes in the connections between neurons. This occurs most prominently in the hippocampus, a brain structure critical for laying down memories for the events of daily life. Thus, during learning, external information must flow to the hippocampus if memories are to be stored.
Once information has been stored in the hippocampus, a different flow of information is required to create a long-lasting memory. During periods of rest and sleep, the hippocampus “reactivates” stored memories, driving activity throughout the rest of the brain. Current theories suggest that the hippocampus acts like a teacher, repeatedly sending out what it has learned to the rest of the brain to help engrain memories in more stable and distributed brain networks. This “consolidation” process depends on the flow of internal information from the hippocampus to the rest of the brain.
Finally, when a memory is retrieved a similar pattern of internally driven flow is required. For many memories, the hippocampus is required for memory retrieval, and once again hippocampal activity drives the reinstatement of the memory pattern throughout the brain. This process depends on the same hippocampal reactivation events that contribute to memory consolidation.
Different flows of information can be engaged at different intensities as well. Some memories stay with us and guide our choices for a lifetime, while others fade with time. We and others have shown that new and rewarded experiences drive both profound changes in brain activity, and strong memory reactivation. Familiar and unrewarded experiences drive smaller changes and weaker reactivation. Further, we have recently shown that the intensity of memory reactivation in the hippocampus, measured as the number of neurons active together during each reactivation event, can predict whether an the next decision an animal makes is going to be right or wrong. Our findings suggest that when the animal reactivates effectively, it does a better job of considering possible future options (based on past experiences) and then makes better choices.
These results point to an alternative approach to helping the brain learn, remember and decide more effectively. Instead of imposing a specific pattern for each experience, we could enhance the flow of information to the hippocampus during learning and the intensity of memory reactivation from the hippocampus during memory consolidation and retrieval. We are able to detect signatures of different flows of information associated with learning and remembering. We are also beginning to understand the circuits that control this flow, which include neuromodulatory regions that are often damaged in disease states. Importantly, these modulatory circuits are more localized and easier to manipulate than the distributed populations of neurons in the hippocampus and elsewhere that are activated for each specific experience.
Thus, an effective cognitive neuroprosthetic would detect what the brain is trying to do (learn, consolidate or retrieve) and then amplify activity in the relevant control circuits to enhance the essential flows of information. We know that even in diseases like Alzheimer’s where there is substantial damage to the brain, patients have good days and bad days. On good days the brain smoothly transitions among distinct functions, each associated with a particular flow of information. On bad days these functions may become less distinct and the flows of information muddled. Our goal then, would be to restore the flows of information underlying different mental functions.
A prosthetic device has the potential to adapt to the moment-by-moment changes in information flow necessary for different types of mental processing. By contrast, drugs that seek to treat cognitive dysfunction may effectively amplify one type of processing but cannot adapt to the dynamic requirements of mental function. Thus, constructing a device that makes the brain’s control circuits work more effectively offers a powerful approach to treating disease and maximizing mental capacity.
Loren M. Frank is a professor at the Center for Integrative Neuroscience and the Department of Physiology at the University of California, San Francisco.
Monday, December 10. 2012
Via Culture Digitally (via Christian Babski)
By Tarleton Gillespie
-----
I’m really excited to share my new essay, “The Relevance of Algorithms,” with those of you who are interested in such things. It’s been a treat to get to think through the issues surrounding algorithms and their place in public culture and knowledge, with some of the participants in Culture Digitally (here’s the full litany: Braun, Gillespie, Striphas, Thomas, the third CD podcast, and Anderson‘s post just last week), as well as with panelists and attendees at the recent 4S and AoIR conferences, with colleagues at Microsoft Research, and with all of you who are gravitating towards these issues in their scholarship right now.
The motivation of the essay was two-fold: first, in my research on online platforms and their efforts to manage what they deem to be “bad content,” I’m finding an emerging array of algorithmic techniques being deployed: for either locating and removing sex, violence, and other offenses, or (more troublingly) for quietly choreographing some users away from questionable materials while keeping it available for others. Second, I’ve been helping to shepherd along this anthology, and wanted my contribution to be in the spirit of the its aims: to take one step back from my research to articulate an emerging issue of concern or theoretical insight that (I hope) will be of value to my colleagues in communication, sociology, science & technology studies, and information science.
The anthology will ideally be out in Fall 2013. And we’re still finalizing the subtitle. So here’s the best citation I have.
Gillespie, Tarleton. “The Relevance of Algorithms. forthcoming, in Media Technologies, ed. Tarleton Gillespie, Pablo Boczkowski, and Kirsten Foot. Cambridge, MA: MIT Press.
Below is the introduction, to give you a taste.
Algorithms play an increasingly important role in selecting what information is considered most relevant to us, a crucial feature of our participation in public life. Search engines help us navigate massive databases of information, or the entire web. Recommendation algorithms map our preferences against others, suggesting new or forgotten bits of culture for us to encounter. Algorithms manage our interactions on social networking sites, highlighting the news of one friend while excluding another’s. Algorithms designed to calculate what is “hot” or “trending” or “most discussed” skim the cream from the seemingly boundless chatter that’s on offer. Together, these algorithms not only help us find information, they provide a means to know what there is to know and how to know it, to participate in social and political discourse, and to familiarize ourselves with the publics in which we participate. They are now a key logic governing the flows of information on which we depend, with the “power to enable and assign meaningfulness, managing how information is perceived by users, the ‘distribution of the sensible.’” (Langlois 2012)
Algorithms need not be software: in the broadest sense, they are encoded procedures for transforming input data into a desired output, based on specified calculations. The procedures name both a problem and the steps by which it should be solved. Instructions for navigation may be considered an algorithm, or the mathematical formulas required to predict the movement of a celestial body across the sky. “Algorithms do things, and their syntax embodies a command structure to enable this to happen” (Goffey 2008, 17). We might think of computers, then, fundamentally as algorithm machines — designed to store and read data, apply mathematical procedures to it in a controlled fashion, and offer new information as the output.
But as we have embraced computational tools as our primary media of expression, and have made not just mathematics but all information digital, we are subjecting human discourse and knowledge to these procedural logics that undergird all computation. And there are specific implications when we use algorithms to select what is most relevant from a corpus of data composed of traces of our activities, preferences, and expressions.
These algorithms, which I’ll call public relevance algorithms, are — by the very same mathematical procedures — producing and certifying knowledge. The algorithmic assessment of information, then, represents a particular knowledge logic, one built on specific presumptions about what knowledge is and how one should identify its most relevant components. That we are now turning to algorithms to identify what we need to know is as momentous as having relied on credentialed experts, the scientific method, common sense, or the word of God.
What we need is an interrogation of algorithms as a key feature of our information ecosystem (Anderson 2011), and of the cultural forms emerging in their shadows (Striphas 2010), with a close attention to where and in what ways the introduction of algorithms into human knowledge practices may have political ramifications. This essay is a conceptual map to do just that. I will highlight six dimensions of public relevance algorithms that have political valence:
1. Patterns of inclusion: the choices behind what makes it into an index in the first place, what is excluded, and how data is made algorithm ready
2. Cycles of anticipation: the implications of algorithm providers’ attempts to thoroughly know and predict their users, and how the conclusions they draw can matter
3. The evaluation of relevance: the criteria by which algorithms determine what is relevant, how those criteria are obscured from us, and how they enact political choices about appropriate and legitimate knowledge
4. The promise of algorithmic objectivity: the way the technical character of the algorithm is positioned as an assurance of impartiality, and how that claim is maintained in the face of controversy
5. Entanglement with practice: how users reshape their practices to suit the algorithms they depend on, and how they can turn algorithms into terrains for political contest, sometimes even to interrogate the politics of the algorithm itself
6. The production of calculated publics: how the algorithmic presentation of publics back to themselves shape a public’s sense of itself, and who is best positioned to benefit from that knowledge.
Considering how fast these technologies and the uses to which they are put are changing, this list must be taken as provisional, not exhaustive. But as I see it, these are the most important lines of inquiry into understanding algorithms as emerging tools of public knowledge and discourse.
It would also be seductively easy to get this wrong. In attempting to say something of substance about the way algorithms are shifting our public discourse, we must firmly resist putting the technology in the explanatory driver’s seat. While recent sociological study of the Internet has labored to undo the simplistic technological determinism that plagued earlier work, that determinism remains an alluring analytical stance. A sociological analysis must not conceive of algorithms as abstract, technical achievements, but must unpack the warm human and institutional choices that lie behind these cold mechanisms. I suspect that a more fruitful approach will turn as much to the sociology of knowledge as to the sociology of technology — to see how these tools are called into being by, enlisted as part of, and negotiated around collective efforts to know and be known. This might help reveal that the seemingly solid algorithm is in fact a fragile accomplishment.
~ ~ ~
Here is the full article [PDF]. Please feel free to share it, or point people to this post.
Monday, March 28. 2011
Via Vague Terrain
-----
by Kevin Hamilton
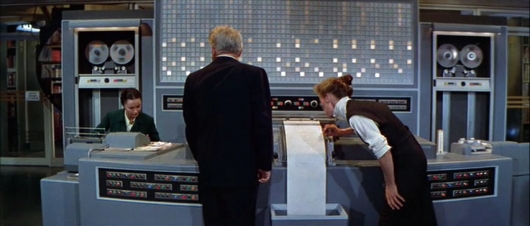
Richard Sumner: Often when we meet people for the first time, some physical characteristic strikes us. Now what is the first thing you notice in a person?
Bunny Watson: Whether the person is male or female.
I followed Watson's debut on Jeopardy about as much as the next guy - the story was unavoidable there for awhile. I read Richard Powers' essay on the pre-paywall version of the New York Times, watched the flashy documentary about Flashy designer Josh Davis, responsible for the avatar seen on screen.
I assumed like others that the AI software was named for Thomas Watson, IBM's founder, or perhaps even for the sidekicks to Alexander Graham Bell or Sherlock Holmes. (Though each of the latter options seemed a mismatch.)
Having finally watched the 1957 film Desk Set, starring Hepburn and Tracy, I think I have found Watson's true origins – in Hepburn's character Bunny Watson.
In the film (adapted from a play), Watson has just returned from a demonstration of the new IBM Electronic Brain (announced by Thomas J. Watson?), to find that her office at a large national television network has been occupied by an IBM "methods engineer" named Richard Sumner (played by Spencer Tracy.) 1
Sumner, who in addition to being a management science expert is an MIT-trained computer engineer, is engaged in a month-long project of studying Watson's office and staff – the Reference Section of the company. Watson and the three women she supervises are the human Google for the company – their phones constantly ring with obscure questions - some of which are so familiar to the women that they can answer without effort, others of which require access to files and books.
Sumner's job, known to us and only suspected and feared by the other main characters, is to design a computer installation for the office. As the company wants some big publicity for this event, Sumner is to keep his mission a secret, leading to greater suspicion on the part of Watson and her team of an impending disaster – would a computer replace their labor?
The film's narrative is anchored by two significant tests. At the beginning, Watson is tested by Sumner, and determined to be a superb computing agent. She is able to count, tabulate, store and recall with uncanny precision, and using counter-rational or supra-rational algorithms. Later, during the story's second big test, the finally installed computer fields some initial queries in its position as reference librarian, and fails.
EMERAC fails because of poor context awareness, something that the mere typist assigned to inputting data doesn't know to compensate for. In the end, EMERAC is only successful - and therefore of value to humanity - when operated by Watson herself, who is able to enter in the right information to makeup for the computer's poor contextual knowledge.
So the conclusion takes us to a happy marriage of computer and operator, in which both are necessary to keeping things running smoothly and efficiently, in the context of a growing world of "big data." (The final problem, and the one we see EMERAC answer correctly, is the question "What is the weight of the Earth?")
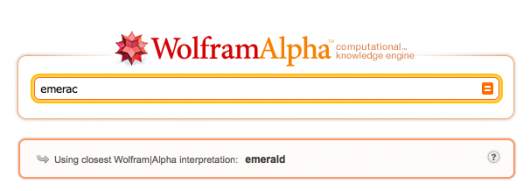
EMERAC is thus more like Wolfram Alpha than the contemporary Watson. The new Watson, named for an operator rather than for a computer, is presented to television viewers as an operator of the Jeopardy interface. (The game is, after all, a button-pushing contest.)
In the new Watson, a man - at least in popular understanding - has replaced a woman at the switch. But perhaps a new configuration of labor has emerged anyway. Consider the change from the former, in which Sumner engineers and maintains the machine in real time, while Bunny operates it, to the newer version, in which multiple sites across multiple temporalities are responsible for the resulting computing event.
Alex Trebeck is in the role of the telephone from Desk Set, merely passing along the queries originating from elsewhere. The Watson AI, dressed in Davis' cartoony dataviz rather than Charles LeMaire's fashions, fields the questions and answers them as a sort of merged operator and machine. Behind the scenes and long before the event, a small army of researchers programmed the AI and fed it data. In Desk Set, this latter job is also visible, through the work of Bunny's staff, who help deliver all the content for the machine to digest.
So with the Jeopardy Watson stunt, we see primarily two changes – a person where a phone used to be, and a machine where there used to be a machine-plus-operator. The sum total of laborers has remain unchanged, though we are less one woman, and plus one man. This cybernetic brain needs no operator, but it does need a user – and it certainly needs an audience.
(1) The whole story takes place at Rockefeller Center and bears many stylistic resemblances to the current NBC sitcom 30 Rock – including a page named Kenneth.
This post was originally published on Critical Commons.
|